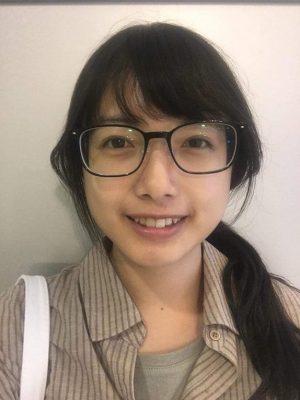
Hsiao-Yu Tung
Carnegie Mellon University
PhD Candidate
Hsiao-Yu (Fish) Tung is a PhD candidate in the Machine Learning Department at CMU, advised by Katerina Fragkiadaki. She is interested in building machines that can understand and interact with the world with minimum human labels. Her research spans across unsupervised learning, computer vision, graphics, robotics, and language. Her research is supported by Yahoo InMind fellowship.
She received her M.S. in CMU MLD and B.S. in Electrical Engineering from National Taiwan University. During her master degree, she worked with Professor Alex Smola on spectral method for Bayesian models and had designed efficient and provable algorithms for unsupervised topic discovery.
Research Abstract:
There has been much debate about what is the right output to get when we design a computer vision system, in particular, for embodied agents — machines that move around and carry out actions in the real world. Should it be some human-defined 3D representations such as triangular meshes or 3D point clouds? However, recovering the complete representations of the 3D world is impossible and sometimes unnecessary (e.g., capturing every fur of a rabbit does not help me catch it). Besides, some semantic information (e.g., the rabbit is soft) is dropped. This has led researchers to look into another potential solution: learning a direct mapping from the pixel space to the target action through end-to-end training. However, these methods have not shown until today to generalize across camera viewpoints and to handle cross-object occlusions, and they usually require an impractical number of samples to learn. Instead of choosing one side and closing our eyes to the other, we argue that methods combining the advantages from the two sides are needed.
I propose learnable 3D representations and put them as the bottleneck of neural network architectures. The 3D bottleneck learns features that encode geometry and semantics and is end-to-end trainable for a downstream task. Using the representation, we learn a model that can predict RGB images from novel views (self-supervised task), detect and segment objects in 3D, and learn action-conditioned object dynamics for action planning. Besides, we show the possibility to discover novel objects in 3D using the learned representation. The proposed 3D-aware latent representation serves as a potential solution towards building machines that learns to see without a teacher, just like humans.