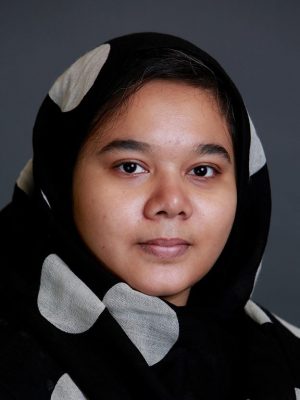
Saima Siddiqui
Argonne National Laboratory
Postdoctoral Associate
Saima Siddiqui has recently joined as a postdoctoral associate at University of Illinois at Urbana Champaign. After completing her PhD in Electrical Engineering from MIT in 2018, she spent nine months at Argonne National Laboratory as a postdoctoral researcher in Materials Science division. Her research is focused to explore novel physical phenomena of electron’s spin and implementing them to building Boolean (logic gates i.e. AND, OR etc.) and non-Boolean devices (i.e. Synaptic and thresholding devices for artificial neural network) for next generation energy-efficient computer architectures. She received her Master of Science degree in Electrical Engineering from MIT in 2014 and completed her undergraduate studies in Electrical and Electronic Engineering at Bangladesh University of Engineering and Technology (BUET) in 2011. After her undergrad, she worked as a lecturer in the same department at BUET. During her PhD, she was involved in organizing Path of Professorship – a workshop for female graduate students and postdocs interested in career at academia.
Research Abstract:
Spintronics promises intriguing device paradigms where electron spin is used as the information token instead of its charge counterpart. Spin based devices are the most promising options for non-volatile memory, beyond-CMOS, in memory computing and neuromorphic accelerators. In the future cognitive era, nonvolatile memories hold the key to solve the bottleneck in the computational performance due to data shuttling between the processing and the memory units. The application of spintronic devices for these purposes requires versatile, scalable device design that is adaptable to emerging material physics.
I have demonstrated spin orbit torque induced magnetic domain wall devices as the building blocks (i.e. linear synaptic weight generator and the nonlinear activation function generator) for in-memory computing, in particular for artificial neural networks using a theory/experiment co-design approach. Spin orbit torque (SOT) driven magnetic tunnel junctions show great potential as energy efficient emerging nonvolatile logic and memory devices. In addition to its energy efficiency, I have taken advantage of the SOT induced domain wall motion in magnetic nanowires to demonstrate the linear change in resistance of the synaptic devices. Modifying the SOT from a heavy metal or utilizing the size dependent magnetoresistance of tunnel junctions, I also have demonstrated a nonlinear activation function for thresholding signals (analog or digitized) between layers for deep learning. The analog modulation of resistances in these devices requires characterizing the resolution of the resistance. To identify the fundamental information limit, I have studied the spatial resolution of discrete magnetic domain wall positions in nanowires.