Computational Biology Session
9:00am-12:00pm, February 23 on Zoom
With the increase in the availability of biological data, computational tools are becoming increasingly important to life sciences. These tools range from signal processing and machine learning for modeling to algorithms and computing systems for decision making. The session will bring together researchers and students to discuss the latest advances in computational biology.
The Computational Biology session taking place on 23rd February from 9:00 am to 12:00 pm will have a keynote speech by Prof. W. Evan Johnson from Boston University. This session will consist of topics including but not limited to the following – (1) computational techniques for biomedical image reconstruction, processing and analysis, (2) computational approaches to structural biology, genomics and neuroscience, and (3) interdisciplinary solutions for bioimaging and life sciences integrating multiple computational approaches and/or hardware and experiments.
Keynote Speaker – Prof. W. Evan Johnson, Boston University
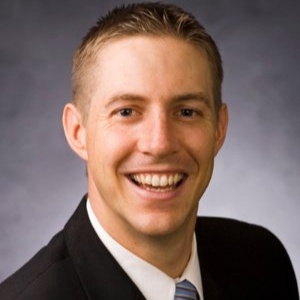
“Host Microbiome Interaction”
Time: 9:50 am – 10:50 am, February 23
Talk Abstract: Big data technologies are playing an increasingly influential role in multiple sectors within industry and research. This is providing significant opportunities for individuals with expertise in the acquisition, management, and analysis of data—so called data scientists. In the biomedical arena in particular, data science and informatics have revolutionized discoveries in molecular research, public heath, and clinical care. One unique application of big data technologies is the use of RNA-sequencing to evaluate the interplay between the microbiome and host immune and inflammatory pathways, particularly in relation to human pulmonary diseases. Here I will discuss several relevant case studies and to demonstrate the value of host-microbe profiling in asthma, lung cancer and tuberculosis research.
Invited Research Speaker – Octavian Ganea, MIT CSAIL

“AI for Drug Discovery”
Time: 9:10 am -9:50 am, February 23
Talk Abstract: Understanding 3D structures and interactions of biological nano-machines, such as proteins or drug-like molecules, is crucial for assisting drug and therapeutics discovery. A core problem is molecular docking, i.e., determining how two proteins or a protein and a drug-molecule attach and create a molecular complex. Having access to very fast computational docking tools would enable applications such as fast virtual search for drugs inhibiting disease proteins, in silico molecular design, or rapid drug side-effect prediction. However, existing computer models follow a very time-consuming strategy of sampling a large number (e.g., millions) of molecular complex candidates, followed by scoring, ranking, and fine-tuning steps. In this talk, I will show that geometry and deep learning (DL) can significantly reduce the enormous search space associated with the docking and molecular conformation problems. I will present my recent DL architectures, EquiDock and EquiBind, that perform a direct shot prediction of the molecular complex, and GeoMol, that models molecular flexibility. I will argue that the governing laws of geometry, physics, or chemistry that naturally constrain these 3D structures should be incorporated in DL solutions in a mathematically meaningful way. I will explain our key modeling concepts such as SE(3)-equivariant graph matching networks, attention keypoint sets, optimal transport for binding pocket prediction, and torsion angle neural networks. These approaches reduce the inference runtimes of open-source or commercial software from tens of minutes or hours to a few seconds, while being competitive or better in terms of quality. Finally, I will highlight a number of exciting on-going and future efforts in the space of artificial intelligence for structural biology and chemistry.
Bio: Octavian Ganea is a postdoctoral researcher at CSAIL-MIT working with Tommi Jaakkola and Regina Barzilay on AI solutions for drug discovery and structural biology using geometric and physical inductive biases. He is part of the Machine Learning for Pharmaceutical Discovery and Synthesis consortium, the Abdul Latif Jameel Clinic for Machine Learning in Health, the DARPA Accelerated Molecular Discovery program, and the ELLIS society. Octavian received his PhD from the Data Analytics Lab at ETH Zurich under the supervision of Thomas Hofmann working on non-Euclidean representation learning for graphs, hierarchical data, and natural language processing. More information: https://people.csail.mit.edu/oct
Student Speakers
Grant Greenberg

“Joint embedding of single-cell multi-modal data”
Time: 10:50 am – 11:10 am, February 23
Single-cell genomics has greatly advanced our knowledge of cell biology. Recent technological innovations now allow for joint measurement of multiple biological data within an individual cell, including RNA gene expression (scRNA), DNA accessibility (scATAC), and surface protein markers (ADT). Such multi-modal technologies provide a valuable new source of information at a single-cell resolution, and give rise to interesting challenges. Many computational methods have been proposed to integrate the disparate data types for applications such as inferring gene regulation, jointly embedding cellular states, and predicting unmeasured modalities. In this work, we describe a deep learning framework to represent the disparate data modalities in a shared latent space. Our model utilizes a variational auto-encoding structure and is designed to account for technical effects in the data. Moreover, we focus on the interpretability of our model based on its design from first principles. We present the results of applying the model on several comprehensive datasets composed of scATAC and scRNA data, or ADT and scRNA data.
Ishita Jain

“Mechanistic modelling of Notch and Biomechanical Cues in Patterned Liver Differentiation”
Time: 11:10 am – 11:30 am, February 23
The bipotential liver progenitors can differentiate into both hepatocytes and biliary cell fates. Previously, we have shown that bipotential liver progenitor cells cultured on a defined circular geometry demonstrate patterned differentiation. The peripheral cells differentiate into biliary cells, while the cells in the interior differentiate into hepatocytes. Here, liver progenitor cell differentiation patterning was used as a model to systematically evaluate the complex interplay of cellular mechanics and Notch signaling along with identifying key combinatorial mechanisms guiding progenitor fate. A hybrid approach of computational modelling along with experiments was used to create perturbations to the differentiation pattern. For the computational model, a set of ODEs based on the Collier Model was simulated for cells on a circular lattice. Further, in vitro and in silico gene knockdowns were created, informing intercellular communication mechanisms mediated by Notch ligands and E-Cadherin. Furthermore, extrinsic perturbations using growth factor treatments both in silico and in vitro systems were performed. Overall, the perturbations in silico and in vitro revealed the spatial control of mechanotransduction-associated components, key growth factor and Notch signaling interactions, and point towards a possible role of E-Cadherin in translating intercellular mechanical cue gradients to downstream Notch signaling.
Soutick Saha
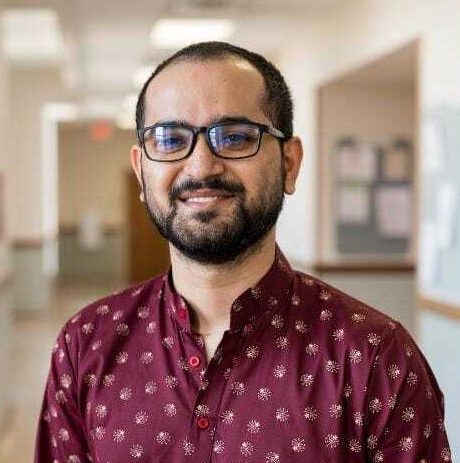
“Inference of signaling mechanism from cellular responses to multiple cues”
Time: 11:30 am – 11:50 am, February 23
Cell signaling networks are complex and often incompletely characterized, making it difficult to obtain a fundamental picture of the mechanisms they encode. Mathematical modeling of these networks provides important clues, but the models themselves are often complex, and it is not always clear how to extract falsifiable predictions. Here we take an alternative approach, using experimental data at the cell level to infer the minimal mechanism that must be encoded in the signaling network. We focus on cells’ response to multiple cues, specifically on the surprising case in which the response is antagonistic: the response to multiple cues is weaker than the response to the individual cues. We systematically build candidate signaling networks one node at a time, using the ubiquitous ingredients of (i) up- or down-regulation, (ii) molecular conversion, or (iii) reversible binding. In each case, our method reveals a minimal, interpretable signaling mechanism that explains the antagonistic response. Our work provides a systematic way to infer molecular mechanisms from cell-level data.
CONTACT US
For more information, please contact the session chair, Anurendra Kumar.